Unlocking the Power of Image Classification with Innovative Labeling Tools
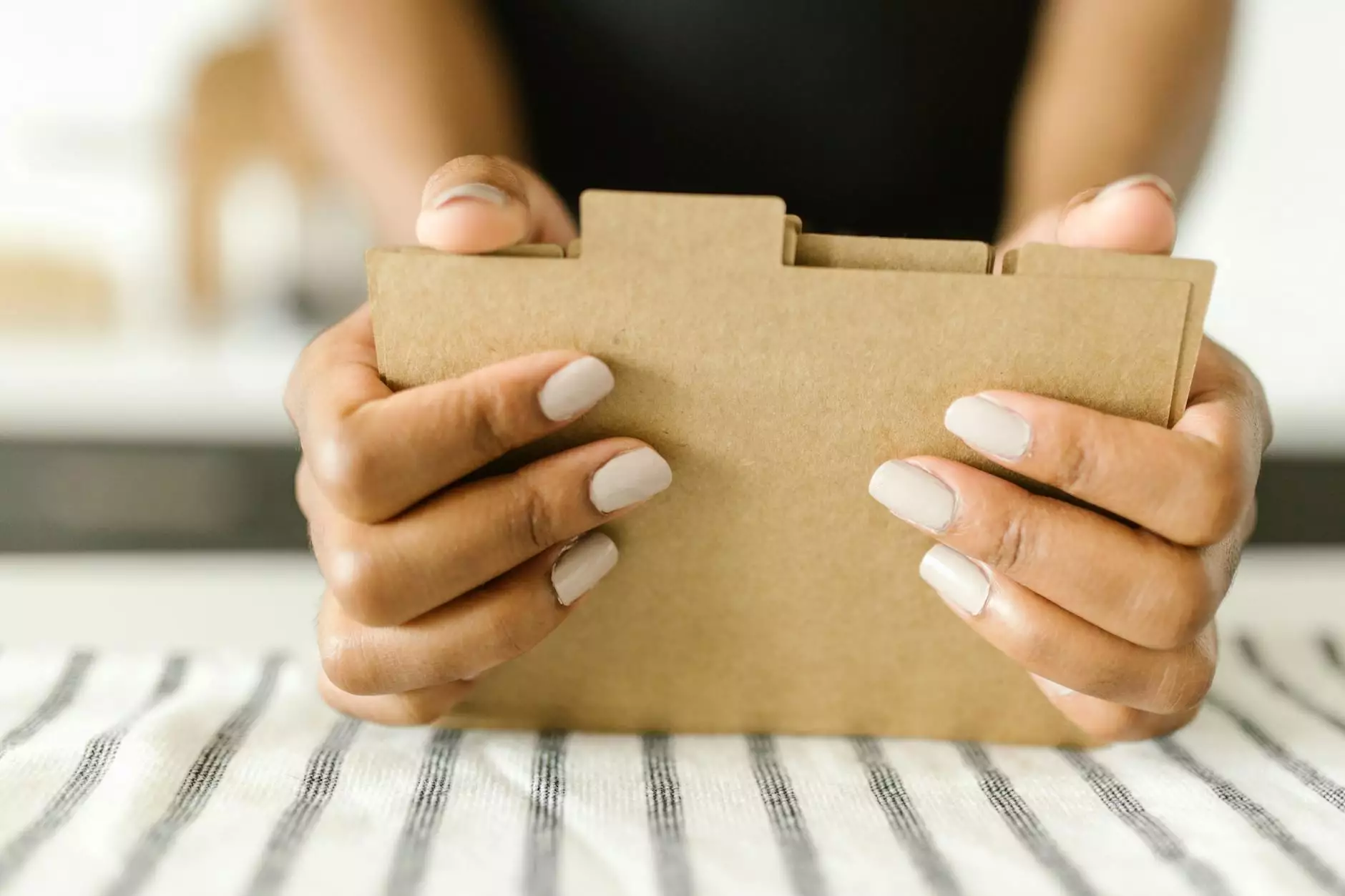
In the rapidly evolving realm of artificial intelligence (AI) and machine learning (ML), the significance of high-quality data is paramount. At the core of this data quality lies the process of careful data annotation, specifically through efficient and effective image classification labeling tools. These tools not only streamline the annotation process but also enhance the accuracy and utility of machine learning models.
Understanding Image Classification
Image classification is a critical machine learning task that involves categorizing images into predefined classes based on their content. This process is essential for various applications, including facial recognition, object detection, and medical imaging. The effectiveness of any classification algorithm hinges on the quality of the training data, which is where image classification labeling tools come into play.
The Importance of Data Annotation in AI
Data annotation serves as the foundation for training algorithms. When it comes to image classification, having accurately labeled datasets drastically improves the performance of AI models. Without precise and relevant annotations, even the most sophisticated algorithms struggle to make correct predictions. Thus, employing a dedicated data annotation platform equipped with comprehensive image classification labeling tools is crucial.
Key Features of Image Classification Labeling Tools
When evaluating image classification labeling tools, it is essential to understand the features that contribute to their efficacy. Some of the prime features include:
- User-Friendly Interface: An intuitive design that allows users to easily navigate through images and labeling options enhances productivity.
- Support for Various Formats: The ability to handle multiple image formats (JPEG, PNG, etc.) ensures versatility in data processing.
- Automated Labeling: Advanced tools incorporate machine learning algorithms to suggest labels, speeding up the annotation process significantly.
- Collaboration Features: Tools that facilitate teamwork allow multiple annotators to work concurrently, ensuring faster project completion timelines.
- Integration with Machine Learning Frameworks: Seamless integration with popular AI frameworks fosters a smooth workflow from data annotation to model training.
Comparing Different Image Classification Labeling Tools
With a myriad of image classification labeling tools available, selecting the right one can be challenging. Here’s how some leading platforms stack up against one another:
1. Keylabs.ai
Keylabs.ai offers robust data annotation tools designed specifically for enhancing image classification tasks. Its cloud-based platform provides scalable solutions, featuring both manual and automated annotation capabilities.
2. Labelbox
Labelbox is another popular choice, known for its highly customizable workflows. Users can create a tailored annotation experience that aligns with the specific needs of their tasks.
3. Amazon SageMaker Ground Truth
AWS SageMaker Ground Truth stands out with its pay-as-you-go pricing model, making it accessible for businesses of all sizes. Its unique capabilities for crowd-sourced labeling can provide a diverse perspective on image categorization.
Benefits of Using Image Classification Labeling Tools
Employing image classification labeling tools brings forth numerous advantages that can transform how AI practitioners approach data preparation:
- Enhanced Efficiency: Automated features reduce the manual workload, allowing teams to focus on refining models rather than spending excessive time on labeling.
- Improved Accuracy: Well-structured labeling processes minimize errors inherent in manual annotations, leading to better-performing ML models.
- Time Savings: Rapid annotation enables quicker turnaround times for projects, empowering businesses to iterate and innovate faster.
- Scalability: Platforms that support large-scale data projects ensure that as your dataset grows, your annotation processes can adapt without compromising quality.
Challenges in Image Classification Annotation
Despite the advantages, there are challenges to overcome in the image classification labeling process:
- Quality Control: Ensuring consistent label quality across large datasets can be difficult, leading to a need for robust review systems.
- Complexity of Images: Some images may contain ambiguous or overlapping classes, complicating the labeling process and potentially introducing errors.
- Resource Intensive: Data annotation can be resource-heavy, requiring significant human input, especially for large-scale projects.
Best Practices for Effective Image Classification Labeling
To maximize the returns on investment for image classification labeling tools, consider the following best practices:
- Define Clear Guidelines: Establish comprehensive guidelines for annotators to follow, ensuring that everyone interprets labels consistently.
- Utilize Automated Assistance: Leverage automated labeling options to speed up the process, while retaining human oversight for final quality assurance.
- Conduct Regular Training Sessions: Keep your annotators well-trained and updated on new labeling practices or techniques.
- Implement Feedback Loops: Introduce mechanisms for annotators to receive feedback on their work to improve future labeling accuracy.
The Future of Image Classification and Data Annotation
As technology advances, the future of image classification and data annotation holds exciting promise. Here are a few trends to watch:
- Increased Use of AI: More sophisticated algorithms will not only improve the speed of annotation but also enhance accuracy through continuous learning from feedback.
- Greater Automation: Expect more robust automated solutions that reduce the need for manual intervention, making the process even more efficient.
- Integration with Augmented Reality (AR): Combining AR with annotation tools may provide immersive ways to label images, especially in fields like gaming and virtual training.
Conclusion
In conclusion, the landscape of AI and machine learning is significantly influenced by the quality of data utilized in training algorithms. Leveraging advanced image classification labeling tools not only streamlines the annotation process but also paves the way for high-performing models. Businesses aiming to excel in AI must invest in the right data annotation platform to ensure that they can handle the scale and complexity of contemporary data demands.
By adopting best practices and staying abreast of industry trends, organizations can harness the full potential of their projects, bolstering their growth and maintaining a competitive edge in the market. With platforms like Keylabs.ai, the possibilities for innovation through meticulously annotated data are boundless.